Computational Mechanics & Scientific Artificial Intelligence Lab (CoMSAIL) focuses on developing the next-generation computational modeling & simulation capability for various computational mechanics problems by integrating multi-resolution data, classic numerical solver, and deep neural networks based on differentiable programming, Bayesian learning/optimization, GPU computing, and uncertainty quantification.
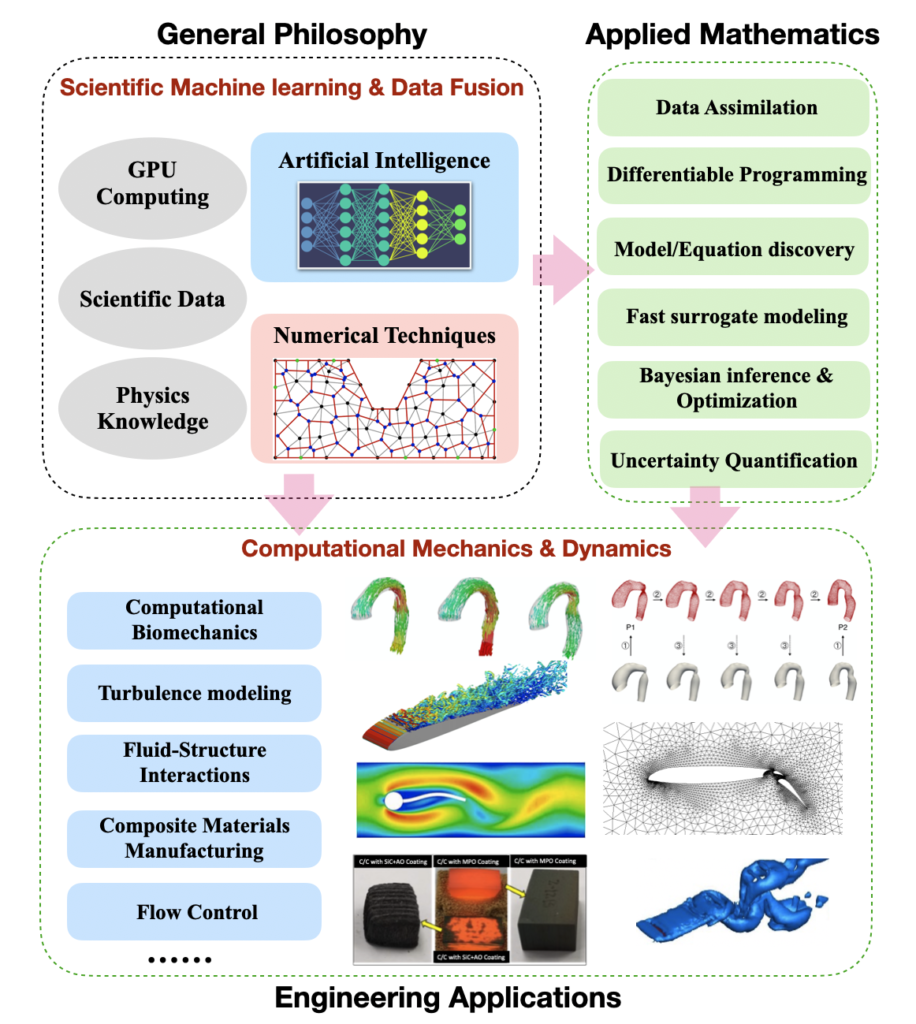
National Science Foundation
- CAREER: Forward and Inverse Uncertainty Quantification of Cardiovascular Fluid-Structure Dynamics via Multi-fidelity Physics-Informed Bayesian Geometric Deep Learning (NSF OAC, 2021 – 2026), $528K, PI.
- OAC Core: A Machine Learning Assisted Visual Analytics Approach for Understanding Flow Surfaces (NSF OAC, 2021-2024), $536K, Co-PI.
- Physics-Constrained Deep Learning for Surrogate Modeling of Dynamics of Fluids and Fluid-Structure Interaction (NSF CMMI, 2019-2022), $300K, PI
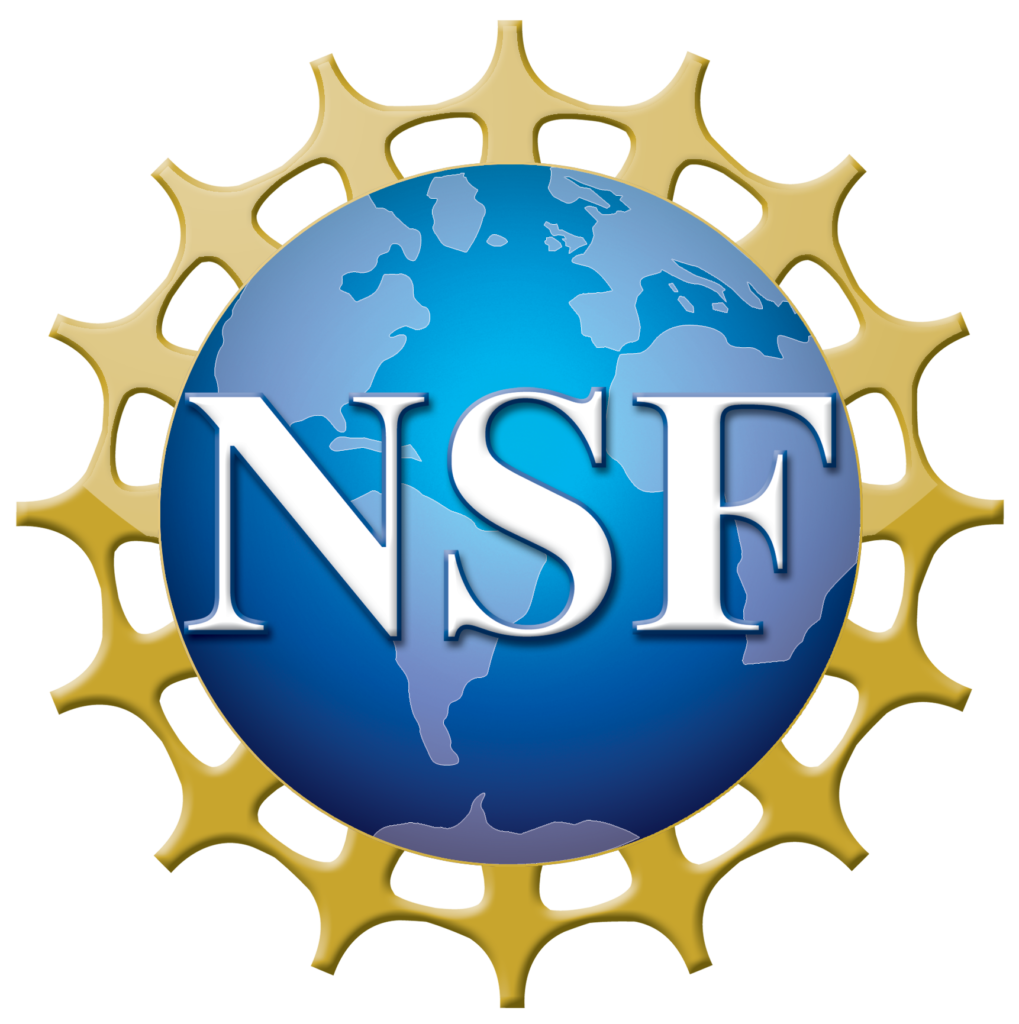
Office of Naval Research (ONR)
- Cost Reduction for Electrochemical Machining Through Hybrid Reduced Order Simulation (STTR, 2023 – 2024), $120K, PI
- Young Investigator Program (YIP) Award: Physics-Preserved Neural Differentiable Computing for Predictive Modeling of Rough-Wall Turbulence. (ONR YIP, 2022 – 2025), $750K, PI
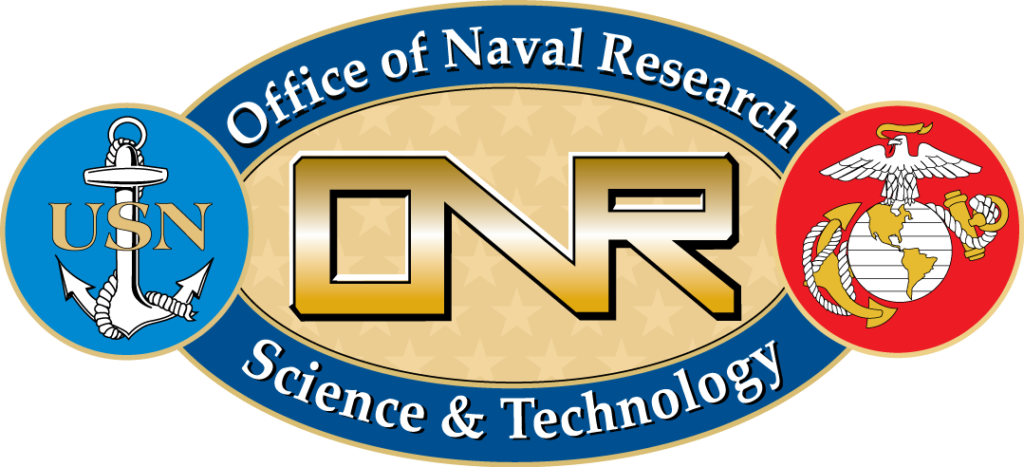
Air Force Office of Scientific Research
- Optimizing Carbon/Carbon Composites Manufacturing by Identifying and Reducing Key Uncertainties for Hypersonic Applications (AFOSR, 2022 – 2025), $1.5M, Co-PI
- DURIPI: Differentiable Neural Computing in Manufacturing Modeling of Hypersonic Materials (AFOSR, 2023-2024) $135K, PI
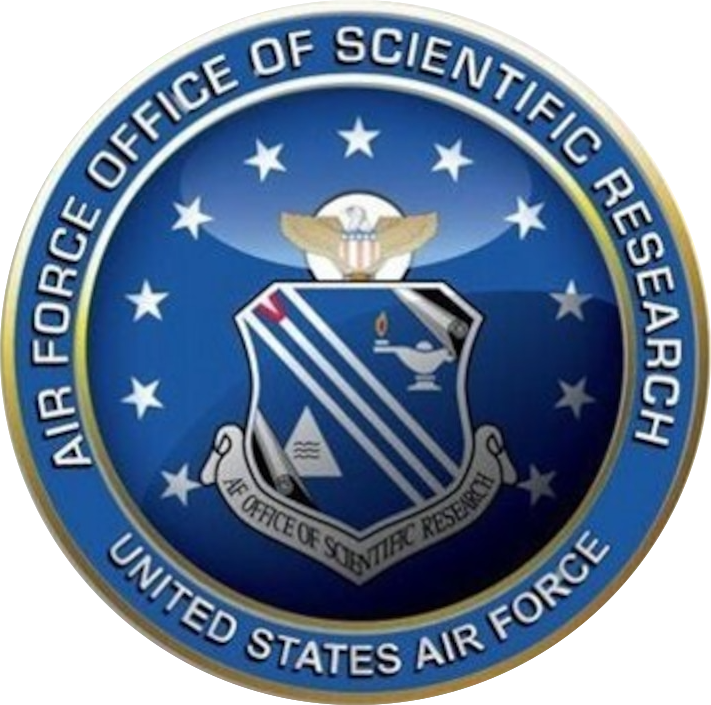
Defense Advanced Research Projects Agency (DARPA)
- Highly Efficient Physics-Informed Deep Learning Model for Nanoscale Transistor Thermal Analysis (DARPA, 2023-2025), $1M, Co-PI
- Physics-Informed Learning for Multiscale Systems (DARPA, 2018 – 2020), $1M, Co-PI
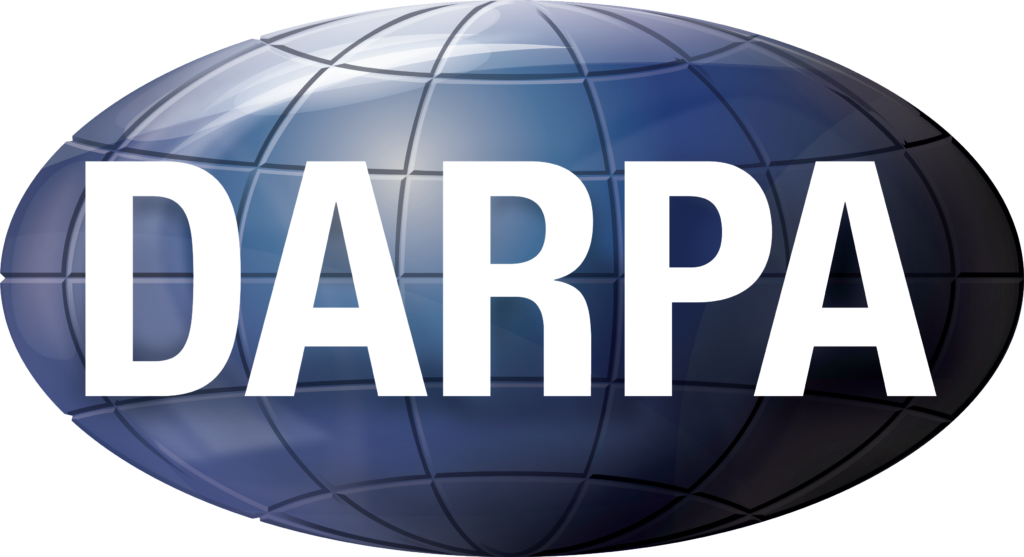
Lucy Family Institute for Data & Society
- Rapid Personalized Image-Based Cardiovascular Flow Modeling Using Bayesian Deep Learning (Lucy, 2022 – 2024), $70K, PI
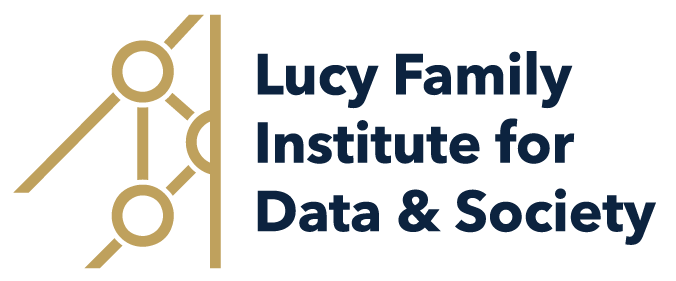
PhysicsX Limited
- AI-enabled predictive model of hemodynamics for a Left Ventricular AssistDevice (LVAD) (2022-2023), $103K, PI
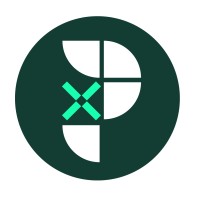
Google LLC
- A Turbulent Inflow Generator with A Data Driven Approach (Google Research Gift), $30K + $24K GCP Credits, PI

ND Energy Center for Sustainable Energy
ND Environmental Change Initiative (ECI)
- Wind Energy Harvesting based on Flow-induced Vibrations and Community Acceptance (Postdoc Fellowship, 2022-2024)
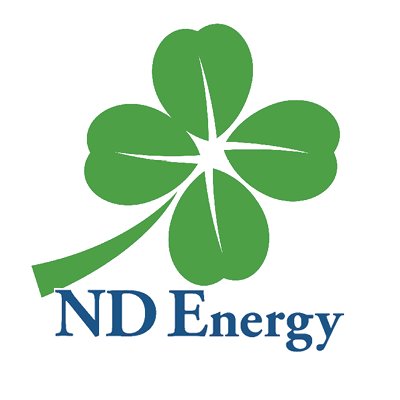
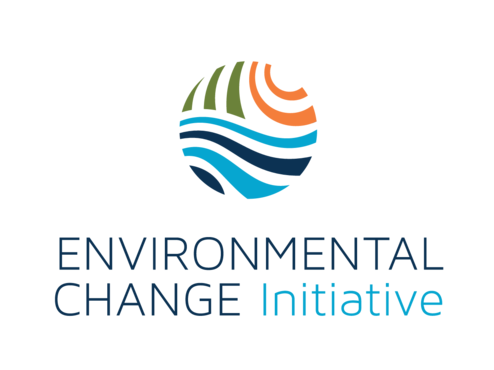
AnalytiXIN
- AnalytiXIN Manufacturing Data Asset Research (AnalytiXIN, 2022-2024), $1.06M, Co-PI
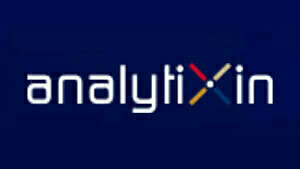