How Streaming Services Automate the Curation of Playlists
by Marcela Wolf Couto
How much of our daily listening is guided by big companies – Spotify, Apple Music, etc? Audio streaming services have transformed the way we consume music, and their recommender systems play a significant role in shaping our listening habits. These systems use complex algorithms to suggest songs, artists, and playlists based on our listening behavior. There is a growing concern that algorithms may only recommend a narrow spectrum of music genres and artists – limiting users’ exposure to a diverse soundtrack. An occurring issue, since a diversified music portfolio can broaden one’s musical horizons by exposing them to sounds they may have not otherwise discovered, leading to a more enriched experience and appreciation for music. So how much control do users really have over their musical experience, and to what extent are their choices influenced by algorithms?
Spotify is the world’s largest music streaming service, but how come more than 480 million people are attracted to its recommender system? Spotify’s algorithm developer, Erik Bernhardsson, explains how this streaming service system is based on user behavior and preferences. A combination of machine learning techniques analyzes listener data and suggests music that is likely to be of interest. This information is combined with the user’s playlist history, the number of times they skip a song, and the length of time they listen to a particular track. This data is then analyzed by a collaborative filtering algorithm — which uses data from other users who have similar listening habits — to make suggestions.
This recommender system is effective, but only for listeners interested in the same genres and not diversifying their musical taste. The personalized approach can result in a feedback loop, where users are presented with recommendations that reinforce their existing preferences and limit their exposure to new genres and artists.
The loop is supported by the use of guided listening and automated curation. Guided listening refers to the way in which audio streaming services present users with curated playlists, that are often categorized by genre or mood; developed by algorithms that analyze users’ listening habits to identify patterns and generate recommendations that are tailored to each individualized preference. Let’s say that you are in the mood for some relaxing music, but you’re not sure what to listen to. You might go to the “Chill” or “Relaxing” playlist curated by the streaming platform, which contains a selection of songs that are known for their soothing and calming qualities – a guided listening in which the platform provides you a pre-made playlist based on your mood. This approach, however, can lead to a suggestion of a narrow spectrum of music, as the algorithms prioritize songs that are similar to those the user has previously listened to. Automated curation, on the other hand, refers to the way in which audio streaming services generate playlists using machine learning. These algorithms analyze vast amounts of data, including songs metadata, user feedback, and external factors such as time of day and location. When you first sign up for Spotify or Apple Music, the platform asks you to select artists and genres that you like, and based on that, it will recommend songs that it thinks you’ll enjoy. Your location is also used as a means to suggest songs that are contextually appropriate and relevant in the environment you currently are – an automated curation where the platform uses data to create personalized recommendations. But this approach can also lead to listeners being presented with a limited selection of music, as the algorithms prioritize songs that are likely to be appealing based on previous listening history.
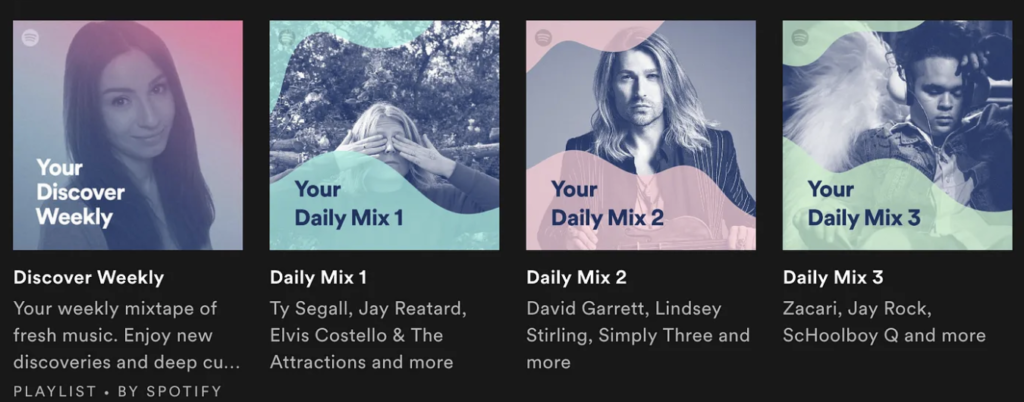
Keeping users from diversifying listening habits is not the only issue within recommender systems: algorithms may also not be fair to emerging artists in the industry. Since these musicians have fewer streams and less data available, they may be overlooked by the recommender systems in favor of more well established artists. As a consequence, the same popular performers are recommended over and over again; monopolizing the industry, thus limiting users’ freedom of choice. In 2019, the British artist Jade Bird, criticized Spotify’s algorithm for failing to include her music in their popular playlists despite having a significant number of streams – likely because her career has not yet reached a well-known parameter. Such matter makes it difficult for new artists to gain exposure on the platform. Another example is the controversy surrounding leading streaming services, where Spotify and Apple Music have been accused of being dominated by major record labels. Arising concerns that the algorithms may be favoring certain artists and genres over others, which may be limiting the diversity of music that is suggested.
Not only that, but there have also been cases where emerging artists have used various tactics to manipulate the algorithms to gain more exposure. Musicians have resorted to buying streams or using fake profiles to artificially inflate their play counts, skewing the system in their favor. While these tactics are unethical and can result in penalties from audio platforms, they highlight the importance of fair and transparent algorithms that are designed to promote diversity and equity in music recommendation.
Additionally, recommender systems are often designed with a narrow focus on user needs, rather than taking into account broader social and cultural factors – language, religion, ethnicity, etc. The algorithms prioritize songs that are likely to appeal to individual tastes rather than promoting cultural exchange. In 2020, for instance, Spotify faced backlash from artists and listeners alike for allegedly promoting the same popular songs on its playlists, leading to accusations of bias towards major labels and established artists. The algorithm was accused of promoting music based on its commercial success, rather than promoting underrepresented artists. This controversy led to the “Loud and Clear” campaign, in which artists called for more transparency within compensation for their art. Another example is the issue surrounding the “Discovery Weekly” playlist on Spotify, which has been accused of promoting formulaic music and not introducing users to new musicians – all illustrations of the consequences promoted by guided listening and automated curation.
Artistic innovation is essential for a thriving music scene, as it encourages experimental and new sounds that push the boundaries of what is possible in music. Consumer demand, on the other hand, plays a significant role in determining which artists are successful, as listeners ultimately decide who they want to support. By promoting competition and preventing monopolistic behavior, antitrust laws can help ensure that a diverse range of artists and genres have a fair chance of success. This can help the promotion of innovation and creativity in the music industry, while also providing consumers with a wider range of options to choose from.
While audio streaming services have revolutionized the way we listen to music, their recommender systems may have unintended – and sometimes intended – consequences. The use of deep learning algorithms within the music industry can lead to guided listening and automated curation. The narrow focus on user needs and poor consideration for social and cultural factors can lead to a lack of variation. Such issues raise important questions about the ethics of music streaming platforms. As we increasingly rely on these services to discover new songs, it is crucial that companies take steps to address these concerns and ensure fairness in the industry.