https://sites.google.com/usacm.org/uq-mlip/home
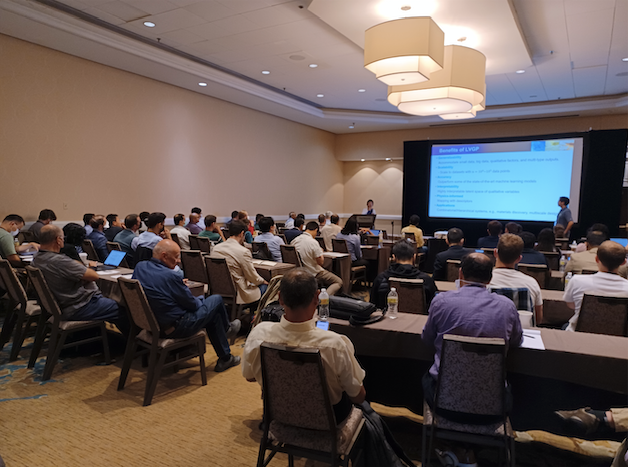
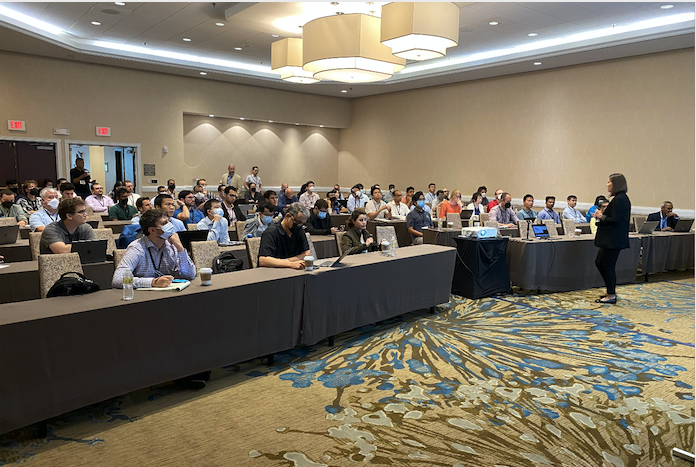
We got one-year research grant from Lucy Institute of Data Science and Society.
Jian-Xun Wang, Assistant Professor in the Department of Aerospace and Mechanical Engineering (AME), and Chaoli Wang, Professor in CSE, were awarded this grant to develop a novel Bayesian deep learning framework for automating vessel segmentation from medical images to enable rapid construction of personalized, patient-specific computational models.
Thank Lucy Institute!
We are welcoming submission of original research to an article collection entitled “Physics-informed Machine Learning and Its Real-world Applications” of Scientific Reports (Springer Nature). As Guest Editor for the Collection, I hope you will consider this excellent outlet for your research in this field.
This article collection is a great opportunity to highlight this important area, and we hope you will be able to contribute. Please don’t hesitate to contact me if you have any questions. The deadline is Nov. 30, 2022. For more details, please see https://www.nature.com/collections/hdjhcifhad/guest-editors
Happy to be selected to the list of 2022 Top 10 Outstanding Chinese American Youth, during Asian American and Pacific Islander Heritage Month.
I will give invited talk at #USACM#UQ Virtual Seminar Organized by USACM UQ TTA. (Time: 15:00 PM-16:00 PM (EST US), 5/12/2022. Zoom Link: listed below). The topic is about “how to leverage physics in ML for computational mechanics — Physics-informed, PDE-structure preserved Learning for problems with complex geometries”. I am happy to have Prof. Michael Brenner be my discussant sharing his great insights in the field of scientific machine learning #SciML. Also thank the organization committee: Abani Patra, Serge Prudhomme, Johann Guilleminot.
In this talk, I will discuss our recent developments of on SciML from several different aspects, including (1) how to leverage physics to inspire network architecture design (PDE-preserved deep learning #PPNN), (2) use physics to inform network training #PINN, (3) physics-informed geometric deep learning (#GeometricDL) for complex geometries and irregular domains. This work is supported National Science Foundation (NSF)#OAC#CMMI, Aerospace & Mechanical Engineering at Notre Dame, Center for Sustainable Energy at Notre Dame, ND-ECI, ND Lucy Institute of Data Science.
Welcome to join us at https://lnkd.in/gH6AHp3V. See you on Thursday
Here is the talk recording
Together with Yeonjong Shin (Brown University) and Xueyu Zhu (U Iowa), we will co-host a 3-part mini-sympoiusm entitled “Recent Advances in Machine Learning and Data-Driven Methods for Physical Sciences and Engineering”, where 12 speakers will give talks on scientific machine learning and data-driven modeling.
Our group will give 5 talks at SIAM UQ22.
Happy to share our work “Predicting Physics in Mesh-Reduced Space with Temporal Attention”, which has been accepted to ICLR 2022. This is a collaborative work with Tufts and DeepMind. Particularly congrats to the student authors X. Han and H. Gao. Arxiv link: https://lnkd.in/dxvZRXWC.
Our article “L. Sun, J.-X. Wang, Physics-constrained bayesian neural network for fluid flow reconstruction with sparse and noisy data, Theor. App. Mech. Let. 10 (2020) 161-169 ” winning the 2021 Highest Citation Paper Award of Theoretical & Applied Mechanics Letters on January 2022. Congrats to Luning!