Pan Du won poster award @ Fall Symposium of Lucy Institute of Data Science and Society. Congrats! The Mayor of South Bend presented the award.
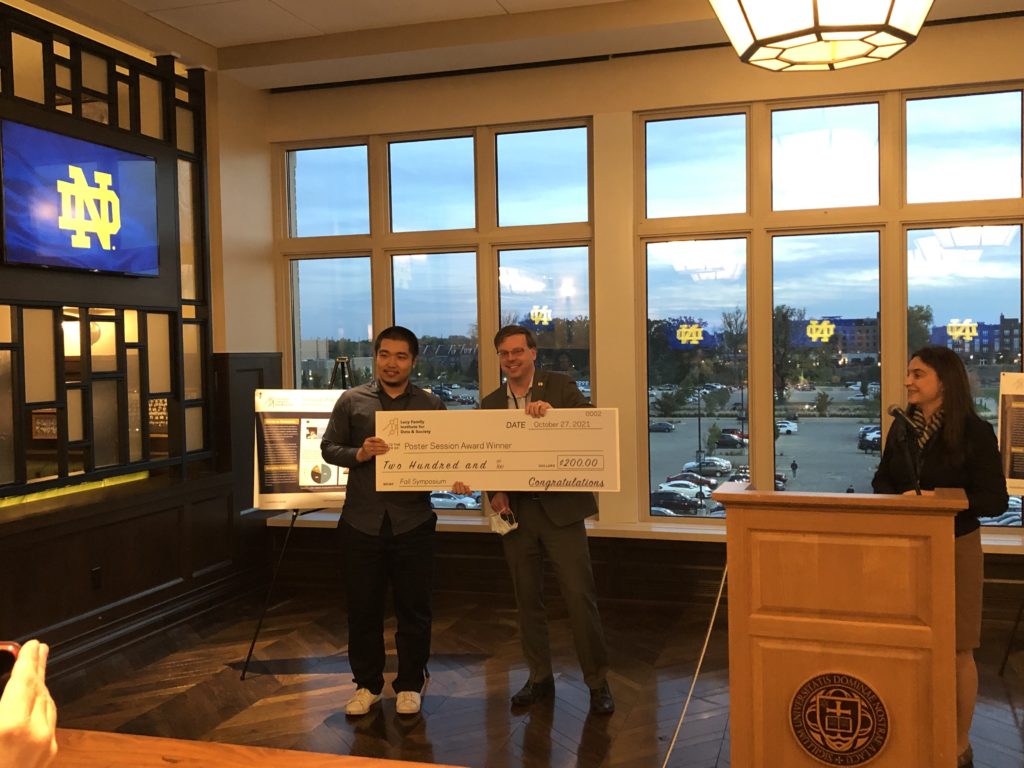
Pan Du won poster award @ Fall Symposium of Lucy Institute of Data Science and Society. Congrats! The Mayor of South Bend presented the award.
Together with Danial, Kathryn, Alireza, and Hao, we will co-host a mini-sympoisum entiled Physics-Informed Learning and Data-Enabled Predictive Modeling and Discovery of Complex Systems, where 15 speakers will give excellent talks on scientific machine learning and data-driven predictive modeling. (10:00-18:00 (UTC), 07/26/2021). Please check out.
Our group will also give 3 talks at USNCCM:
Together with Prof. Huan Xun@University of Michigan, we will host a two-section mini-symposium (MS48) entiled: Physics-aware machine learning for solving and discovering PDEs, part I (MS48) and part II (MS105)
Part I (MS 48), Tuesday, July 20
Part II (MS 105), Friday, July 23
Congrats to Pan Du on his first publication in PLoS One
I will organize a mini-symposium entitled “Physics Informed Learning for Modeling and Discovery of Complex Systems” Parts I and II on 03/03/2021 at SIAM CSE. Moreover, our group will also give several talks at CSE21.
MS Talk: Wang et al. Physics-Informed Discretization-Based Learning: a Unified Framework for Solving PDE-Constrained Forward and Inverse Problem (2:15-2:30 CST, 03/03/2021) https://meetings.siam.org/sess/dsp_talk.cfm?p=108358
MS Talk: Han et al. Suppreresolution and Denoising of Flow Imaging using Physics-Constrained Discrete Learning (4:35-4:50 CST, 03/01/2021) https://meetings.siam.org/sess/dsp_talk.cfm?p=108020
MS Talk: Sun et al. System Identification by Sparse Bayesian Learinng (5:35-5:50 CST, 03/04/2021) https://meetings.siam.org/sess/dsp_talk.cfm?p=108437
Poster: Pan et al. Patient-Specific CFD Modeling of Aortic Dissection Augmented with 4D Flow MRI https://meetings.siam.org/sess/dsp_talk.cfm?p=110813