Dr. Zhang is a Leonard C. Bettex Collegiate Professor of Computer Science at University of Notre Dame, where she is leading a Machine Intelligence and kNowledge Engineering (MINE) group.
Her research broadly addresses ways that enable computer machines to learn by the use of diverse types of data. Specifically, she is interested in designing machine learning algorithms for learning from complex and large-scale streaming data and graph data, with applications to recommendation systems, knowledge discovery, and natural language understanding. Her recent research notably advances the application of AI in scientific disciplines such as Chemistry, Biology and Physics. More information can be found in the publications grouped by research problems, or the full list of over 200 peer-reviewed papers.
She was invited to deliver an Early Career Spotlight talk at IJCAI-ECAI 2018. In 2009, she was awarded the European Research Consortium for Informatics and Mathematics (ERCIM) Alain Bensoussan Fellowship. She regularly serve on the Program Committee for premier conferences like SIGKDD (Area Chair, Senior PC), AAAI (Area Chair, Senior PC), IJCAI (Area Chair, Senior PC), etc. She also serves as Editor-in-Chief of ACM SIGKDD Explorations, associated editor for IEEE Transactions on Dependable and Secure Computing (TDSC) and Information Sciences.
Prior to joining the University of Notre Dame, she was an Associate Professor in Computer Science at KAUST, Saudi Arabia. She completed her Ph.D. degree in computer science from INRIA-University Paris-Sud, France, in July 2010. She received her master and bachelor degrees from Xi’an Jiaotong University, China.
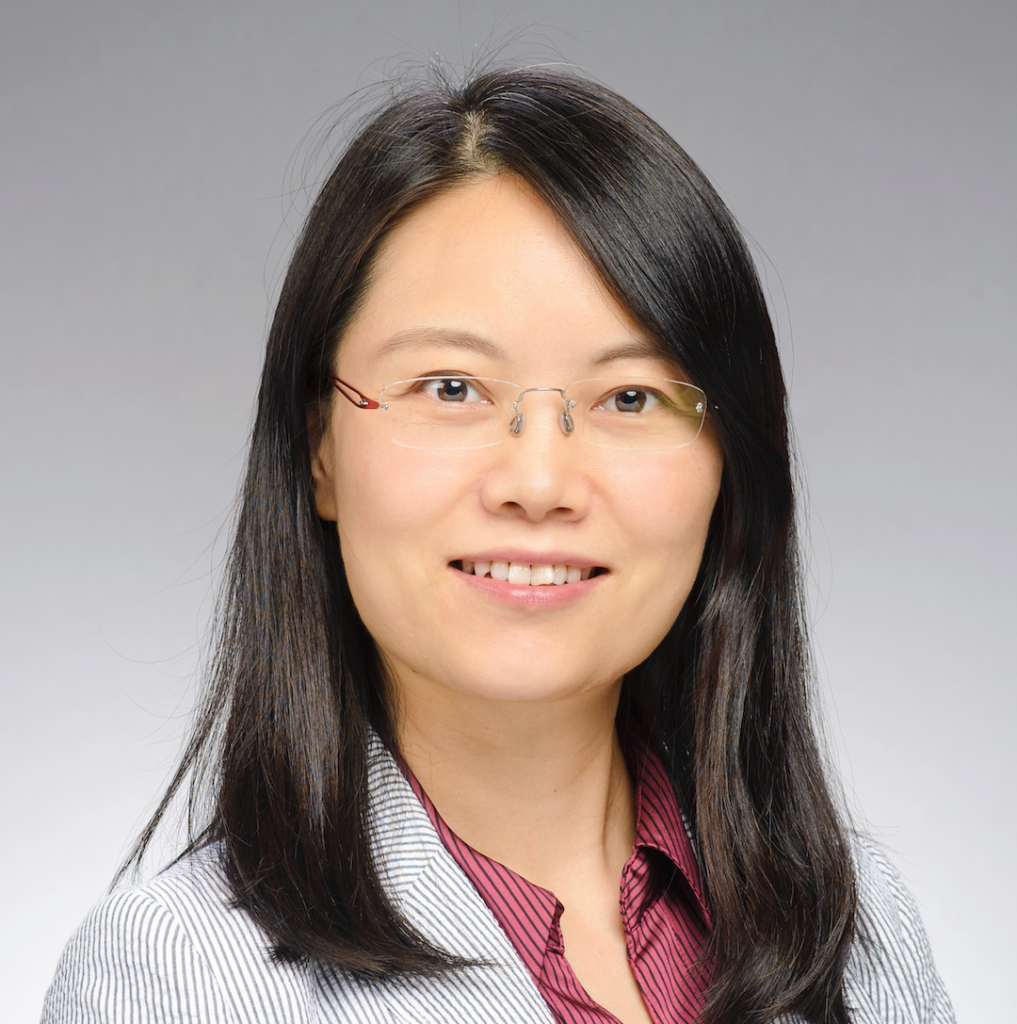
Latest News
- May 2024
- Congratulations to Ziyi! The 15th PhD graduated from our lab, with his thesis “Exploring Trustworthy Concerns in Computer Vision: From Deterministic To Generative Domains“.
- Our recent work regarding LLMs and Trustworthy AI
- Congratulations to Yue Huang, who will join us in August very soon, for his ICML 2024 paper: TrustLLM: Trustworthiness in Large Language Models.
- Congratulations to Taicheng, for his IJCAI 2024 survey paper: Large Language Model based Multi-Agents: A Survey of Progress and Challenges.
- Congratulations to Zhenwen, for his ACL 2024 paper: SceMQA: A Scientific College Entrance Level Multimodal Question Answering Benchmark
- Congratulations to Yujun, for his ICML 2024 paper: Attack-free Evaluating and Enhancing Adversarial Robustness on Categorical Data
- October 2023
- Congratulations to Zhenwen! Two of his recent papers, focused on solving Math Word Problems, have been accepted for EMNLP 2023. Check this one: Let GPT be a Math Tutor: Teaching Math Word Problem Solvers with Customized Exercise Generation, and the other one entitled “UniMath: A Foundational and Multimodal Mathematical Reasoner” will be online soon.
- September 2023
- PI Dr. Zhang is awarded another NSF grant (1.5 million US dollars) for the research project of Exploiting Federal Data and Beyond: A Multi-modal Knowledge Network for Comprehensive Wildlife Management under Climate Change.
- Do you know what LLMs models can do in chemistry? Check our recent paper accepted by NeurIPS 2023 Datasets and Benchmarks track. It offers an extensive benchmark across eight varied tasks, and delves into the potential and constraints of LLMs in addressing chemical tasks. Congratulations to Taicheng and Kehan for spearheading this pivotal work!
- August 2023
- PI Dr. Zhang is awarded an NSF grant (1 million US dollars) for the research project of Cybertraining for Chemical Data scientists, abbreviated as C2D.
- Call for papers!
- Dr. Zhang is the co-chair of WSDM’24 Demo (Due: Sep 24, 2023). Accepted papers will be included in the WSDM’24 proceedings.
- Dr. Zhang is the co-chair of User Modeling and Recommendation track at The Web Conference 2024 . (Due: Oct 5 (abstract), Oct 12 (full-paper), 2023)
- Dr. Zhang is the Editor-in-Chief of SIGKDD Explorations (the December 2023 issue is accepting submission of surveys, news, opinion papers, vision papers and so on).
- November 2022
- We have 5 papers accepted by AAAI 2023! Congratulations to Zhenwen, Qiannan, Hongyan, Xiuying and Xiaoting! These papers present our recent study of data-efficient learning from graphs, trustworthy machine learning and natural language understanding. More can be found here.
- August 2022
- NSF Center for Computer-Assisted Synthesis led by Prof. Olaf Wiest is funded! In the 5-year project, PI Dr. Zhang’s team will work on designing novel machine learning models for chemical research problems. See the news.
- We have another molecular generation paper got accepted by CIKM 2022. It attempts to build an understandable embedding space for molecular generation.
- We have two papers about Recommendation Systems got accepted by CIKM 2022.
- Dr. Zhang is appointed as the Editor-in-Chief of ACM SIGKDD Explorations. More at News.
- May 2022
- Our work of Few-shot Heterogeneous Graph Learning via Cross-domain Knowledge Transfer is accepted by KDD 2022. Anther few-shot learning on graphs survey paper will appear in IJCAI 2022
- Our work of applying PU-learning on graph completion, Positive-Unlabeled Learning with Adversarial Data Augmentation for Knowledge Graph Completion will also appear in IJCAI 2022
- More of our recent work on graph learning can be found at Learning with Graphs.
- January 2022
- Our work of Understanding the Robustness Against Evasion Attack on Categorical Data is accepted by ICLR 2022. More of our work in this topic can be found at Robust and Trustworthy Machine Learning
- Our work of Graph Alignment with Noisy Supervision is accepted by TheWebConf 2022. A related work of handling noisy labels in knowledge graph alignment can be found in our KDD 2020 paper, entitled REA: Robust Cross-lingual Entity Alignment Between Knowledge Graphs.
- December 2021
- Our work of Self-Augmented Graph Contrastive Learning is accepted by AAAI 2022. More of our graph learning work can be found at Learning with Graphs/Networks
- Our work of GNN-Retro: Retrosynthetic planning with Graph Neural Networks is accepted by AAAI 2022. This paper uses GNN to predict the synthesis routes for target molecules. Another related work of molecular graph generation can be found in our CIKM 2021 paper, entitled GF-VAE: A Flow-based Variational Autoencoder for Molecule Generation.
I am looking for well-motivated Ph.D. students to work on machine learning and data mining problems. Please send me your CV if you are interested.