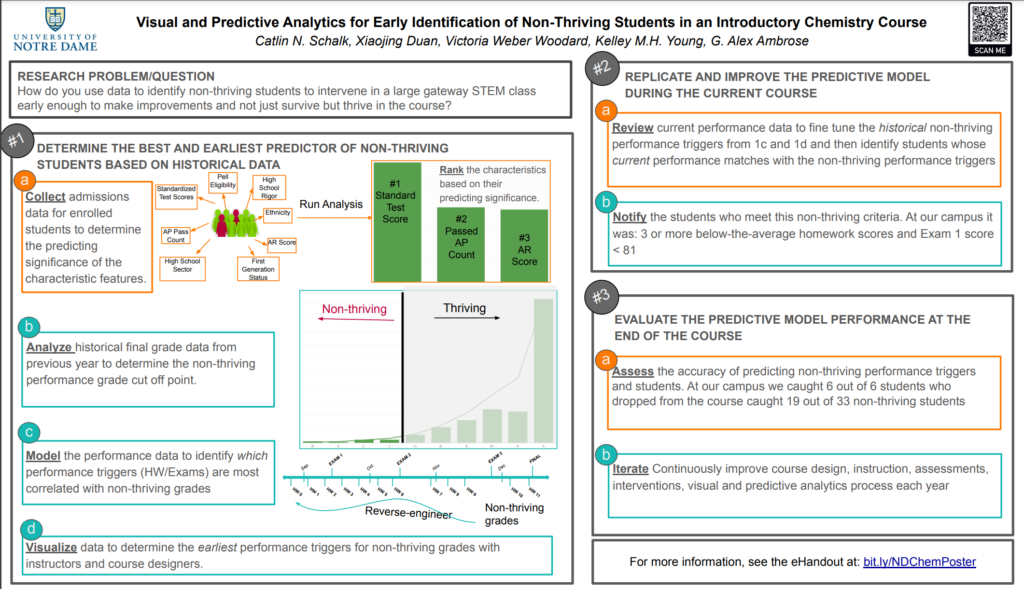
Click here to download or zoom in for a larger view
Citation:
Schalk, Catlin, Young, Kelley, Duan, Xiaojing, Woodard, Victoria Weber, Ambrose, G. Alex (2021) “Visual and Predictive Analytics for Early Identification of Non-Thriving Students in an Introductory Chemistry Course” Midwest Scholarship of Teaching & Learning (SoTL) Annual Conference. Virtual.
Abstract:
We present a data-driven framework for identifying at-risk, or rather “non-thriving,” students in a large-enrollment introductory general chemistry course. This predictive learning analytic methodology was used to identify underperforming students during the early part of the course, through a hybrid approach of statistical modeling and domain expert decision making.
Description:
Background & Lit Review
There has been a long history and large body of knowledge in pursuing our research question in post secondary chemistry education. For most of these studies, the objective was to decrease drop, fail, withdrawal (DFW) rates by classifying “at-risk” predictors and intervening before the identified students began college coursework. These predictors often took the form of cognitive characteristics, such as standardized test scores, university-made placement exams focusing on mathematical ability, prior conceptual chemistry knowledge and logical reasoning skills. (Spencer1996; Kennepohl2010; Wagner2002; Pickering1975; Bird2010; Ozsogomonyan1979). Another less objective area of inquiry was students’ affective experiences which included self-concept, attitude, and motivation (Xu2013; Chan2014; DanielHouse1995), and intervention typically involved remedial courses(Kilner2018, Walmsley1977, Bentley2005; Mason2001; Kogut1993), or preparatory courses and transition programs (Hunter1976; Krannich2977; Shields2012, Stone2018). Previous efforts at our university include identifying non-thriving students during a required first-year-experience (FYE) course (Syed2019), and an introductory engineering course (Bartolini2020).
Research Problem
Although identifying “at-risk” students has been a popular field of research for introductory science courses, we make the distinction between “thriving” and “surviving” because the students identified in the current study are not necessarily at risk of failing the course, but they are likely to withdraw from the course or from their STEM program. How do you identify and intervene students who are not thriving while it is early enough to make improvements in the course?
Research Question
What are the best and earliest predictors of non-thriving learners early in the course, and what data-driven methods can we provide administrators and instructors to identify these students?
Method
Our hybrid approach combined exploratory data analysis to determine potential cut off points for non-thriving triggers through visualized data sets, and supervised machine learning to identify and utilize significant predictive features of students’ course success. Objective quantitative data was coupled with decision-making by domain experts (course professors and coordinators, advisors, data scientists, and learning experts from the university’s teaching and learning center). This modeling and visualization approach ensured campus context was taken into consideration when manipulating this largely data-driven approach. Our statistical analysis, suggested machine-learning models, and interactive visualizations of the multidimensional data sets are described in this section to show how we addressed our research question.
Step 1: Determine the non-thriving point for the final course grade.
1a. Collect historical performance data from the previous year.
1b. Visualize data into a grade distribution chart.
1c. Set the non-thriving point.
Step 2: Determine the best and earliest predictors of non-thriving students based on historical data.
2a. Collect historical performance data from the previous year and identify all non-thriving students.
2b. Model student data to identify the performance features most correlated to non-thriving performance.
2c. Visualize the data to determine the specific cut-off ranges.
Step 3: Replicate and improve the model early on during the current course.
3a. Export gradebook data for the current set of students at the data collection time point
3b. Filter the data to identify the students whose performance matched the predicted trigger for non thriving students from the previous year.
The earliest performance triggers for non-thriving grades ranked greatest to least from left to right.
Results & Conclusion
Student performance data was used to create learning analytics visuals to aid in discovering trends among non-thriving students, while domain experts made decisions about appropriate cut-off points to classify non-thriving performance. With the overall goals of closing opportunity gaps, maximizing all students’ potential for success in the course, and increasing STEM retention rates, we use student admissions and performance data visuals to ultimately create an environment that better supports all first semester chemistry students, and early on in the course.
Previous efforts to increase STEM retention rates have centered around identifying “at-risk” students before the course begins, based on admissions data. In this paper, we expanded the efforts of the current research in two areas: 1) broadening the search criteria to students who are likely non-thriving, not necessarily “at-risk” of failing the course; and 2) utilizing early and current course performance data instead of before-course characteristics. These two focus points allowed us to capture a more refined demographic of students, with the goal of helping all students to not just survive, but thrive in STEM programs. These methods better prepared us to support all students based on their performance in class, not just their before-course attributes, many of which are inherently biased and cannot account for many contextual differences. Additionally, in our approach to organizing data into interactive visual representations, we made our methods accessible to all faculty and administrators so that context-driven decisions can be made for the course.
With a largely data-driven approach, we sought to answer the research question: What are the best and earliest predictors of non-thriving learners early in the course, and what tools can we provide administrators and instructors to identify these students? Through a K- Nearest Neighbor modeling approach with one semester of data, it was determined that the best performance predictors of non-thriving students was 2 or more below-average homework scores, and an exam 1 score below 81. However, using these exact cutoffs did not appear to be the best strategy for identifying students in the following semester. A method of iterative refinement was implemented to update the appropriate selection criteria and will continue to be used until our model is fined tuned.
As we collect data from more semesters, we plan to continue to use of this iterative refinement on our model to determine the best set of assignments and amount of time needed to accurately predict the non-thriving status of our students. The methods we have described here provide scholar-practitioners with a set of tools that can be replicated and customized for STEM courses on their campus. We recognize that different institutions will have different definitions of thriving, and course structures. We provide examples specific to our institution for context, but we encourage those that seek to utilize our method to customize this process to one that fits the specifics of their institution. This will entail using data-driven approaches to identify a thriving cutoff point, suggesting a cutoff date and number of assignments for identifying non-thriving students, and implementing best-practice intervention approaches to offer students non-thriving students a boost.
References
Bentley, A. B.; Gellene, G. I. A six-year study of the effects of a remedial course in the chemistry curriculum. Journal of Chemical Education 2005, 82, 125–130.
Chan, J. Y.; Bauer, C. F. Identifying at-risk students in general chemistry via cluster analysis of affective characteristics. Journal of Chemical Education 2014, 91, 1417–1425.
Daniel House, J. Noncognitive predictors of achievement in introductory college chemistry. Research in Higher Education 1995, 36, 473–490.
Hunter, N. W. A chemistry prep course that seems to work. 1976; https://pubs.acs.org/sharingguidelines.
Kennepohl, D.; Guay, M.; Thomas, V. Using an online, self-diagnostic test for introductory general chemistry at an open university. Journal of Chemical Education 2010, 87, 1273–1277.
Kilner, W. C. ConfChem Conference on Mathematics in Undergraduate Chemistry Instruction: The Chem-Math Project. Journal of Chemical Education 2018, 95, 1436–1437.
Kogut, L. S. A general chemistry course for science and engineering majors with marginal academic preparation. Journal of Chemical Education 1993, 70, 565–567.
Krannich, L. K.; Patick, D.; Pevear, J. A pre-general chemistry course for the under-prepared student. Journal of Chemical Education 1977, 54, 730–735.
Mason, D.; Verdel, E. Gateway to Success for At-Risk Students in a Large-Group Introductory Chemistry Class. Journal of Chemical Education 2001, 78, 252–255.
Ozsogomonyan, A.; Loftus, D. Predictors of general chemistry grades. Journal of Chemical Education 1979, 56, 173–175.
Pickering, M. Helping the high risk freshman chemist. Journal of Chemical Education 1975, 52, 512–514.
Shields, S. P.; Hogrebe, M. C.; Spees, W. M.; Handlin, L. B.; Noelken, G. P.; Riley, J. M.; Frey, R. F. A transition program for underprepared students in general chemistry: Diagnosis, implementation, and evaluation. Journal of Chemical Education 2012, 89, 995–1000.
Stone, K. L.; Shaner, S. E.; Fendrick, C. M. Improving the success of first term general chemistry students at a liberal arts institution. Education Sciences 2018, 8, 5.
Spencer, H. E. Mathematical SAT test scores and college chemistry grades. Journal of Chemical Education 1996, 73, 1150–1153.
Syed, M.; Duan, X.; Anggara, T.; Alex Ambrose, G.; Lanski, A.; Chawla, N. V. Integrated closed-loop learning analytics scheme in a first year experience course. ACM International Conference Proceeding Series. New York, New York, USA, 2019; pp 521–530.
Wagner, E. P.; Sasser, H.; DiBiase, W. J. Predicting students at risk in general chemistry using pre-semester assessments and demographic information. Journal of Chemical Education 2002, 79, 749.
Walmsley, F. A course for the underprepared chemistry student. Journal of Chemical Education 1977, 54, 314–315.
Xu, X.; Villafane, S. M.; Lewis, J. E. College students’ attitudes toward chemistry, conceptual knowledge and achievement: Structural equation model analysis. Chemistry Education Research and Practice 2013, 14, 188–200.
Acknowledgements
Dan Gezelter, Kevin Abbott, and Pat Miller