
Post by Malcolm Itter, a graduate student with Andrew Finley at Michigan State University. Malcolm received an Outstanding Student Paper Award for this work at AGU 2016!
Charcoal particles deposited in lake sediments during and following wildland fires serve as records of local to regional fire history. As paleoecologists, we would like to apply these records to understand how fire regimes, including fire frequency, size, and severity, vary with climate and regional vegetation on a centennial to millennial scale. Sediment charcoal deposits arise from several sources including: 1) direct transport during local fires; 2) surface transport via wind and water of charcoal deposited within a lake catchment following regional fires; 3) sediment mixing within the sample lake concentrating charcoal in the lake center. A common challenge when using sediment charcoal records is the need to separate charcoal generated during local fire events from charcoal generated from regional and secondary sources. Recent work by PalEON collaborators including myself, Andrew Finley, Mevin Hooten, Phil Higuera, Jenn Marlon, Ryan Kelly, and Jason McLachlan applies statistical regularization to separate local and regional charcoal deposition allowing for inference regarding local fire frequency and regional fire dynamics. Here we describe the general concept of regularization as it relates to paleo-fire reconstruction. Additional details can be found in Itter et al. (Submitted).
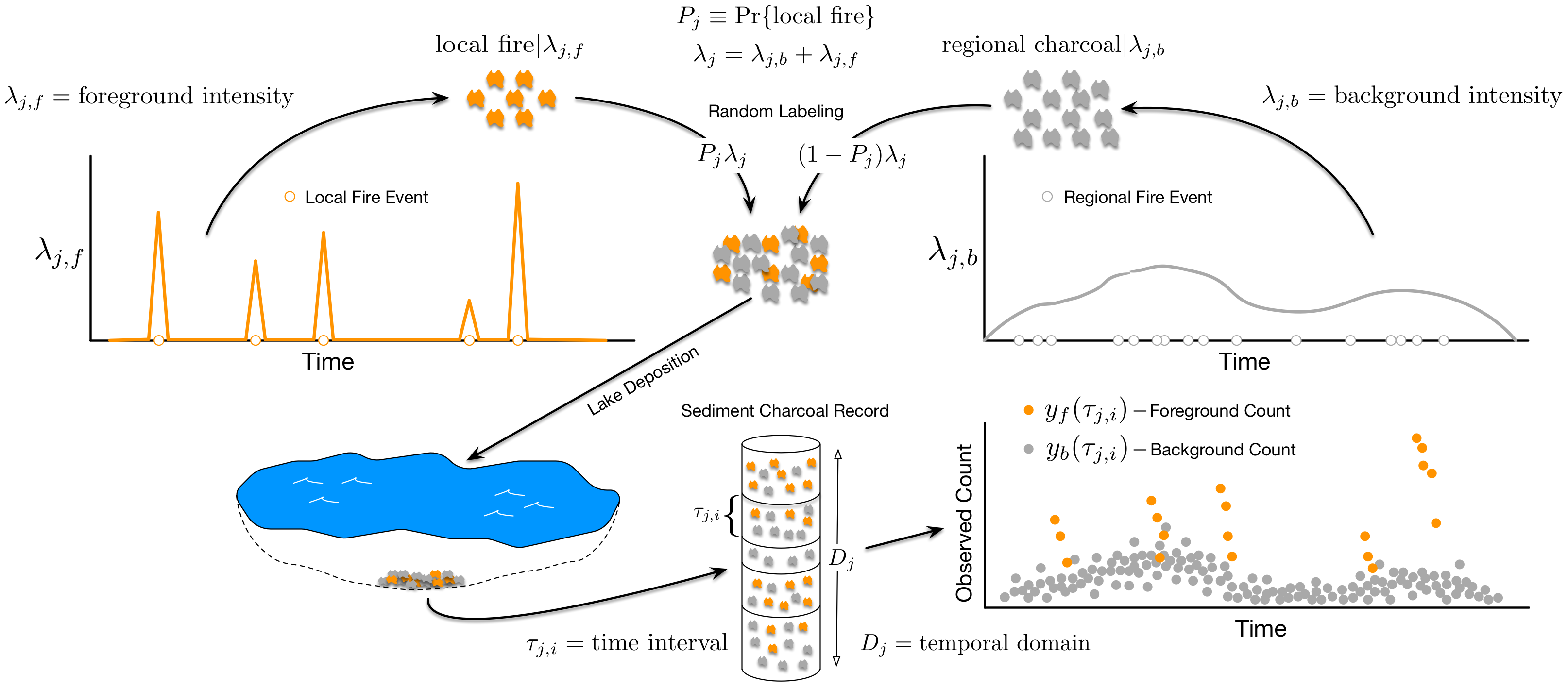
Figure 1: Illustration of theoretical charcoal deposition to a lake if charcoal particles arising from regional fires were distinguishable from particles arising from local fires (in practice, charcoal particles from different sources are indistinguishable). The figure does not depict charcoal arising from secondary sources such as surface water runoff or sediment mixing.
Figure 1 illustrates primary and regional charcoal deposition to a sample lake. We can think of charcoal deposition to a sample lake as being driven by two independent processes in time: a foreground process driving primary charcoal deposition during local fires, and a background process driving regional and secondary charcoal deposition. In practice, charcoal particles arising from different sources are indistinguishable in sediment charcoal records. We observe a single charcoal count over a fixed time interval. Direct estimation of foreground and background processes is not possible without separate background and foreground counts. We overcome the lack of explicit background and foreground counts by making strong assumptions about the nature of the background and foreground processes. Specifically, we assume the background process is smooth, exhibiting low-frequency changes over time, while the foreground process is highly-variable, exhibiting high-frequency changes in charcoal deposition rates associated with local fires. These assumptions follow directly from a long line of paleoecological research, which partitions charcoal into: 1) a background component that reflects regional charcoal production varying as a function of long-term climate and vegetation shifts; 2) a peak component reflecting local fire events and measurement error.
We use statistical regularization to ensure the assumption regarding the relative smoothness and volatility of the background and foreground processes is met. Under regularization, we seek the solution to an optimization problem (such as maximizing the likelihood of a parameter) subject to a constraint. The purpose of the constraint, in the context of Bayesian data analysis, is to bound the posterior distribution to some reasonable range. In this way, the constraint resembles an informative prior distribution. Additional details on statistical regularization can be found in Hobbs & Hooten (2015) and Hooten & Hobbs (2015).
In the context of sediment charcoal records, we model two deposition processes under the constraint that the background process is smooth, while the foreground process is volatile. We use unique sets of regression coefficients to model the background and foreground processes. Both sets of regression coefficients are assigned prior distributions, but with different prior variances. The prior variance for the foreground coefficients is much larger than the prior variance for the background coefficients. The prior variance parameters serve as the regulators (equivalent to a penalty term in Lasso or ridge regression) and force the background process to be smooth, while allowing the foreground process to be sufficiently flexible to capture charcoal deposition from local fires.
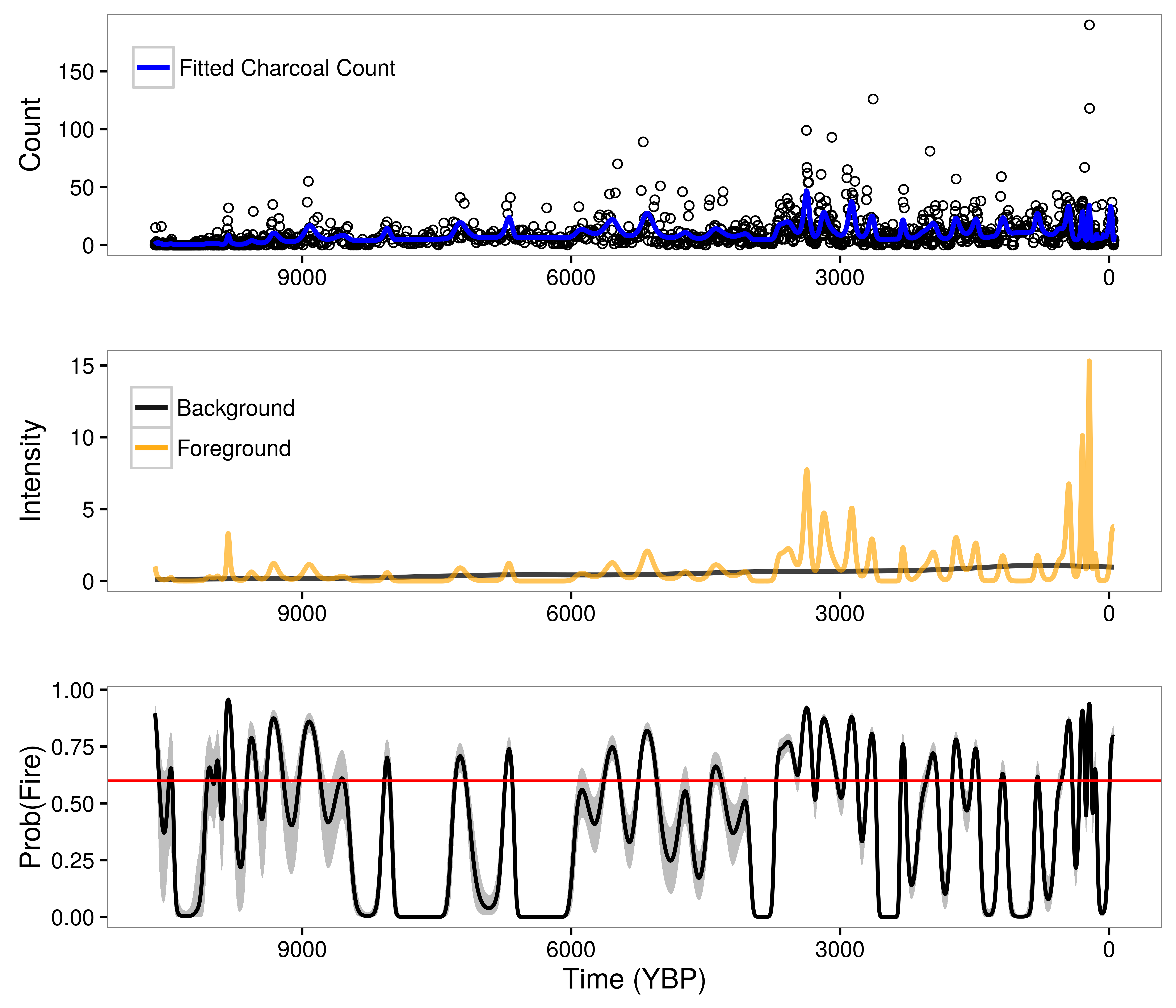
Figure 2: Model results for Screaming Lynx Lake, Alaska. Upper panel indicates observed charcoal counts along with the posterior mean charcoal count (blue line). Middle panel illustrates posterior mean foreground (orange line) and background (black line) deposition processes. Lower panel plots posterior mean probability of fire estimates for each observed time interval (black line) along with the upper and lower bounds of the 95 percent credible interval (gray shading) and an optimized local fire threshold (red line).
Figure 2 shows the results of regularization separation of background and foreground deposition processes from a single set of charcoal counts for Screaming Lynx Lake in Alaska. The probability of fire values presented in the lower panel of Figure 2 follow from the ratio of the foreground process relative to the sum of the background and foreground processes. We would not be able to identify the background and foreground processes without the strong assumption on the dynamics of the processes over time and the corresponding regularization. The benefits of using such an approach to model sediment charcoal deposition are: 1) our model reflects scientific understanding of charcoal deposition to lakes during and after fire events; 2) we are able to identify local fire events from noisy sediment charcoal records; 3) the background process provides a measure of regional fire dynamics, which can be correlated with climate and vegetation shifts over time.
References
1. Hobbs, N.T., Hooten, M.B. 2015. Bayesian Models: A Statistical Primer for Ecologists. Princeton University Press, Princeton, NJ.
2. Hooten, M.B., Hobbs, N.T. 2015. A guide to Bayesian model selection for ecologists. Ecololgical Monographs, 85, 3-28.
3. Itter, M.S., Finley A.O., Hooten, M.B., Higuera, P.E., Marlon, J.R., Kelly, R., McLachlan, J.S. (Submitted). A model-based approach to wildland fire reconstruction using sediment charcoal records. arXiv:1612.02382