Post by Kelly Heilman, a graduate student with Jason McLachlan at the University of Notre Dame
If trees could talk…
Every year, oak trees in the Midwest awaken in spring, to spread their leaves to grow through the summer, and settle down for a long winter’s rest in the fall. As we watch this seasonal cycle, trees warn us of the coming of winter as they shed their leaves, putting on a glorious show of red, gold and orange. At times, trees tell us to slow down by inviting us into their expansive shade to rest, read a book, and listen the birds sing in the distance. However, to many dendrochronologists, trees talk about something else–their records of the past. The patterns of each season’s tree growth are recorded in the annual rings of trees, and are protected like a memory beneath the tree’s bark. These annual variations in tree rings provide researchers with information on the responses of trees to climate variations, as well as to other stresses that trees face, such as competition with their neighbors for light, and fire disturbances. All this makes tree ring records particularly useful tools for looking into the past. So, in a way trees can talk . . . but it takes some effort for us to listen to them.

Trees in the temperate zone, such as this Bur Oak, record annual rings of growth, allowing us to count their rings to get tree age, and correlate growth with climate records. Pictured above is a 5-mm wide core sample from a tree at Bonanza prairie SNA (Scientific and Natural Area) in Minnesota, with the bark pictured on the left and the center of the tree on the right. (Click on image for a larger view.)
Looking at the past responses of ecosystems to climate variations through the lens of tree rings can provide a better understanding of how these ecosystems might respond to future changes. My research focuses broadly on the savanna-forest boundary in the Midwest, both on the environmental conditions that form this boundary and how environmental changes impact these savannas and forests. Over the last century, humans have altered the landscape in the Midwest, through large scale agriculture, land-use change, fire suppression, changes in CO2 and climate shifts (Goring et al. 2016, Rhemtulla et al. 2007). Many of these changes have likely affected the growth, survival, and climate sensitivity of trees, which could impact the trajectory of forests in the future. Therefore, I set out to quantify how savanna and forest trees functioned both in the past, and on the modern landscape. Using annual growth increments recorded in tree rings, my objective was to quantify how both modern and past ecosystems functioned (in terms of how much carbon they uptake and store in their annual growth rings), and to determine if and how tree growth patterns vary across temperature, precipitation, and soil gradients.
Thoughts from the field (don’t forget your bug spray and sunscreen):
To view the annual rings of tree growth, we collected tree core samples from sites across the historic savanna-forest boundary in the Midwest from several Minnesota Scientific and Natural Areas (SNAs), Minnesota State Parks, State Parks in Iowa and Missouri, as well as several sites within McHenry County Conservation District in Illinois (see map). In my sampling design, I aimed to capture the growth responses of young and old trees in both savannas and forests, across the wet-to-dry climate gradient in the Midwest. We targeted several Oak species, including Bur Oak (Quercus macrocarpa), White Oak (Quercus alba), Red Oak (Quercus rubra), and Chinkapin Oak (Quercus muehlenbergii), but also sampled several eastern forest species as well. With the help of fellow Paleonistas (Ann Raiho, Monika Shea) and field technicians Evan Welsh and Santi Thompson, we sampled tree cores at 23 different sites during the summers of 2015 and 2016. Coring trees can be monotonous, physically difficult, and relaxing all at the same time. Lucky for us, we got to go to some beautiful places across the Midwest.

Map of all the sites where we collected tree core samples from during 2015 and 2016. Background color represents mean annual precipitation (MAP) of the region obtained from PRISM climate data. Tree cores were collected from savannas and forests that occur along the historic prairie-forest boundary in the Midwest. (Click on image for a larger view.)

Coring a large Bur Oak tree in a savanna at Maplewood State Park, Minnesota.
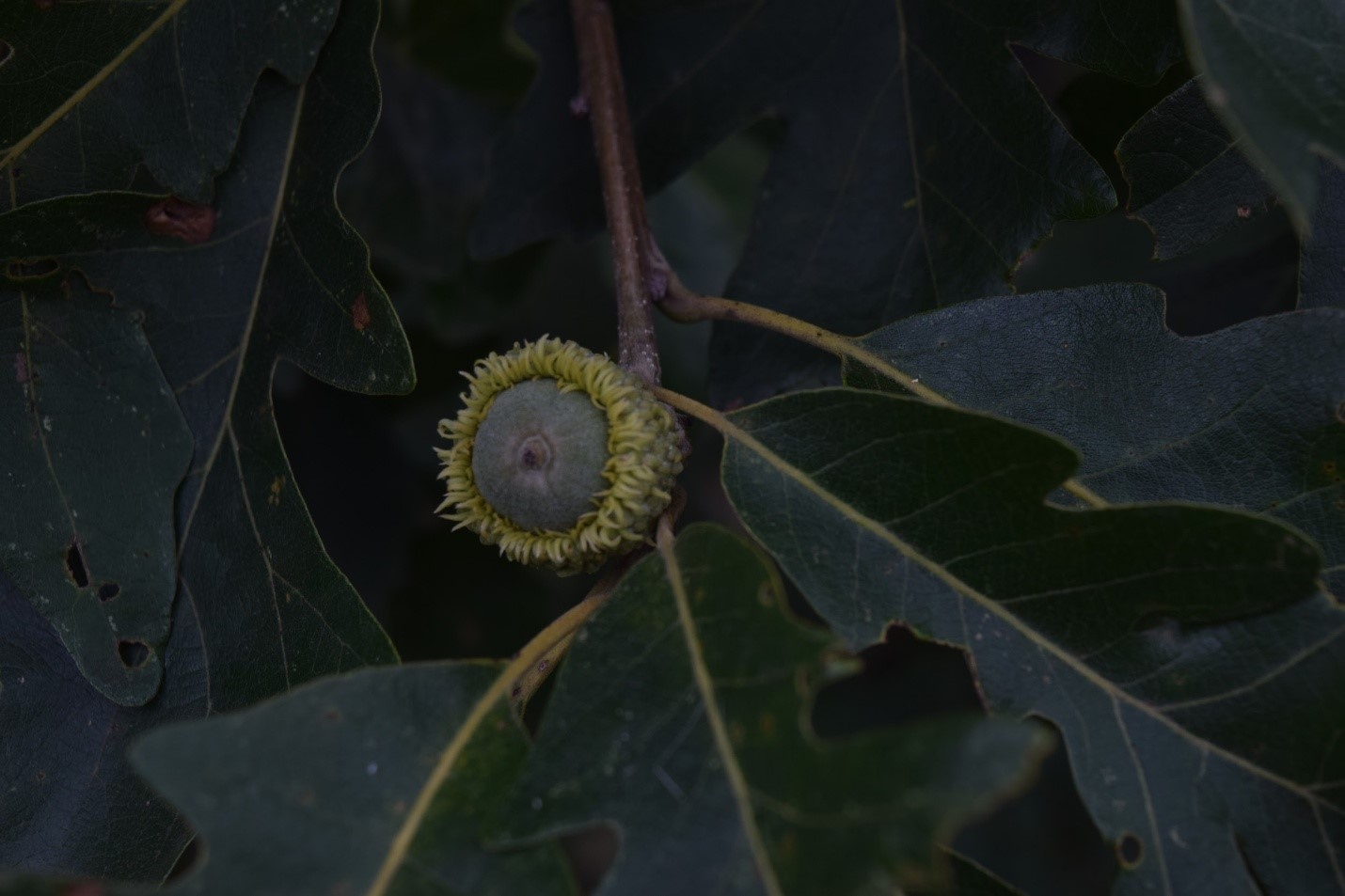
Bur Oak acorn from a young tree located in St. Croix savanna SNA in Minnesota
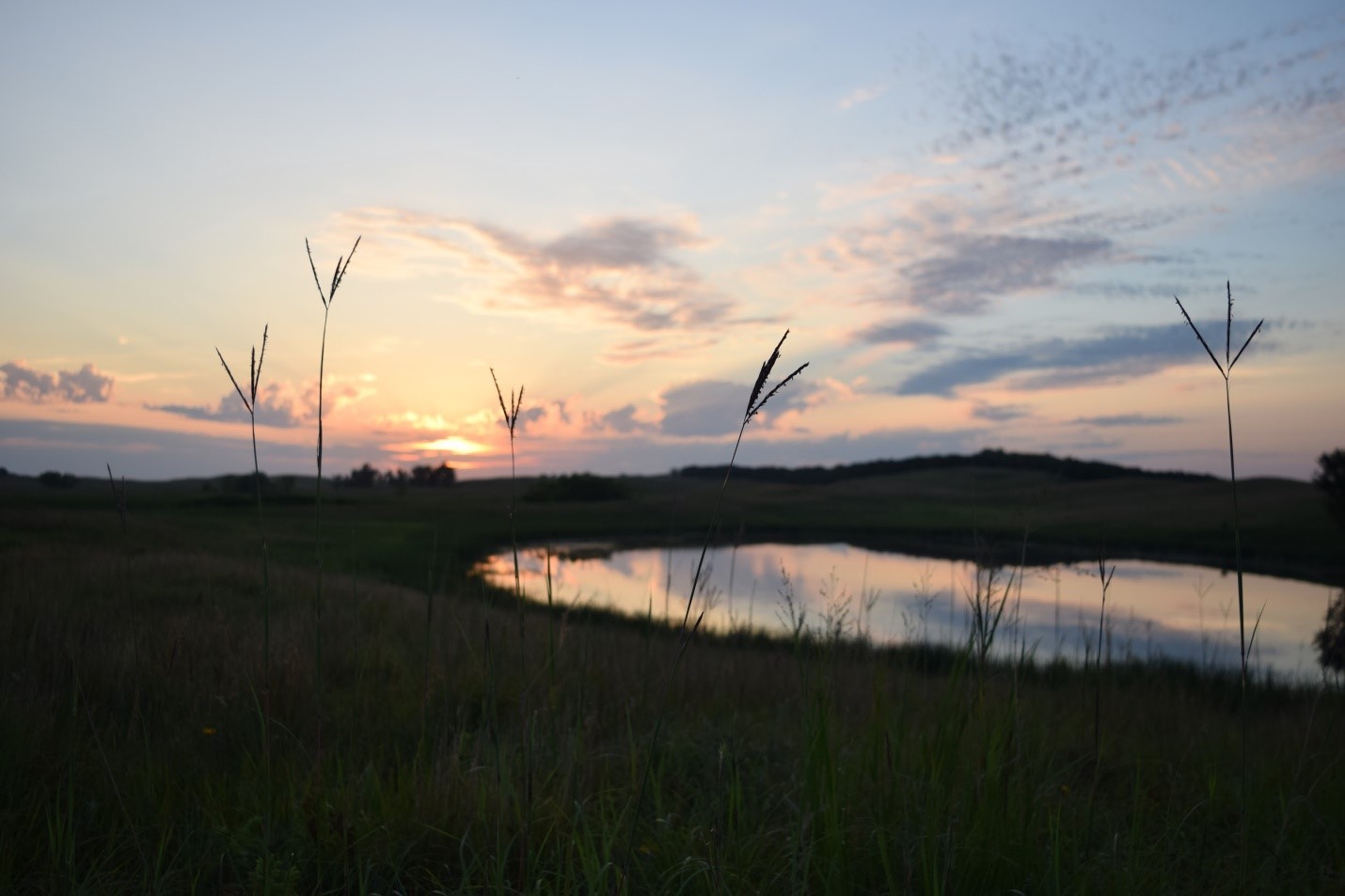
Working out in the field gave us opportunities to see some awesome ecosystems and sunsets. Looking out onto to prairie from a savanna at Glacial Lakes State Park, MN, the tallest plants were not trees, but tallgrass prairie plants, such as the Big Bluestem, or “turkey foot” (Andropodon gerardii) pictured here.

An open savanna and prairie complex at Mound Prairie SNA, in Minnesota.

The Oak savanna canopy is sparse compared to a closed forest, letting in plenty of light for understory grasses and forbs to grow.
After driving a couple thousand miles total, spending over 30 nights in a tent in 2015 and 2016, and battling what seemed like an infinite amount of mosquitoes and ticks, we headed back to the lab to measure the width of each annual tree ring, and determine how climate affects Midwestern oak tree growth.
Back at the Lab:
Once we returned from the field, the cores were glued to wooden mounts, sanded, counted and measured. This work was done with the help of several awesome undergraduate students over the last two years, including: Jacklyn Cooney, Clare Buntrock, Santi Thompson, and Da Som Kim. Once the cores were measured and cross-dated with each other (using common “marker” years of extremely low growth, such as the 1934 Dust bowl drought, to double check our measurements), we have a temporal record of growth fluctuations for each site.
What climate factor affects oak tree growth in savannas and forests?
Tree growth responds strongly to the most limiting factor to their growth.
For example, in water limited regions of Southwestern North America, tree growth is highly correlated with interannual precipitation and drought, often making tree ring records from these regions good candidates for precipitation reconstructions (Charney et al. 2016, Peterson 2014). However, in many Eastern North American forests, water availability for growth is not a huge limiting factor, and tree growth is more sensitive to summer temperatures, drought, and light availability (Peterson 2014, Charney et al. 2016). The savanna-forest boundary in the Midwest is located between these Eastern closed forests and the more water limited prairies to the West. Therefore, tree species that occur along this boundary are often thought to exist at the edge of their theoretical and climatic range boundaries, and theoretically could respond strongly to moisture stress or temperature stress. The historic transition from open prairie to savanna to forests occurred at a range of precipitation and temperature climatic envelopes; this transition zone in Minnesota had low mean annual precipitation (300-600 mm/year), and much higher mean annual precipitation in Indiana & Illinois (700-1200). Therefore, I originally hypothesized that these western savannas and forests may respond more strongly to low precipitation and drought than Eastern savannas and forests.
Contrary to my original hypotheses, oak tree ring growth is not primarily controlled by precipitation in oak trees near the savanna-forest boundary. Rather, tree growth at most sites is strongly linked to summer drought severity and summer temperatures. The negative impacts of drought on tree ring growth are likely mediated by temperature-induced drought stress, as suggested by the strong negative correlations with minimum and maximum June and July temperatures at almost all sites. While growth at some sites is mildly correlated to late summer precipitation, these places tend to have sandy soils, suggesting that future decreases in precipitation could have larger negative consequences for tree growth on sites with sandy soils. Interestingly, despite low moisture availability, sites with the lowest mean annual rainfall were only weakly correlated with monthly precipitation, suggesting that perhaps these systems are relying heavily on deeper groundwater sources for water. However, sites with low rainfall, such as Bonanza Prairie SNA in Minnesota, do have strong sensitivity to drought indices (Palmer Drought Severity Index, PDSI) and temperature indicating that high temperature drought stress, rather than water stress due to low precipitation is more important in this ecosystem.
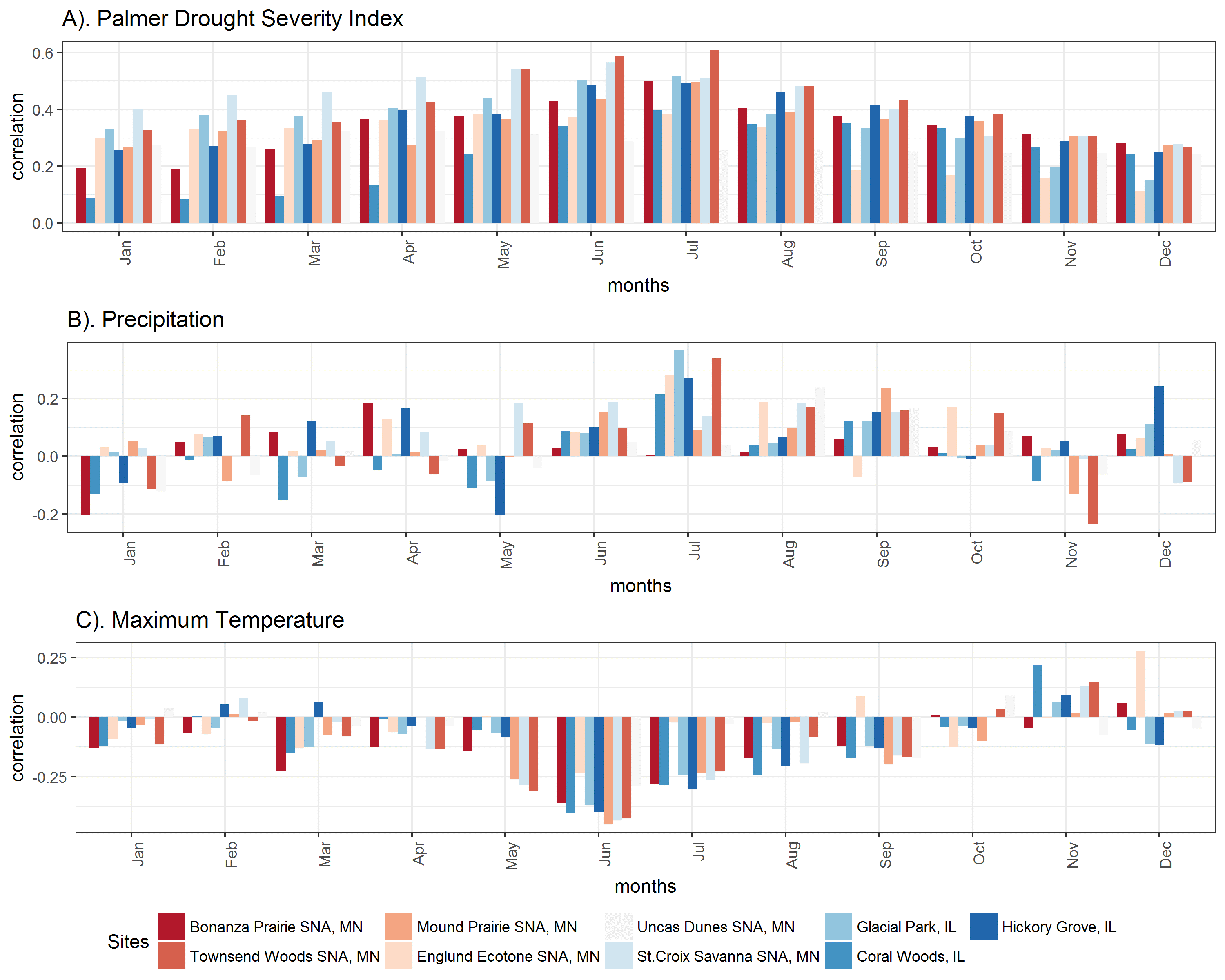
Correlations with monthly climate indicate that Oak trees at most sites are most sensitive to summer drought index and summer temperatures, but few are strongly sensitive to monthly precipitation. Red colored sites have lower mean annual precipitation, and blue sites have higher mean annual precipitation. A). Tree growth at all sites is most strongly correlated to summer drought (Palmer Drought Severity Index is positive in non-drought periods and negative in drought periods). B). July precipitation is only weakly correlated with growth at some sites. C). Tree growth is somewhat negatively correlated with summer maximum temperatures. (Click on image for a larger view.)
Have growth sensitivities changed over time?
The climate-growth relationship is often assumed to be constant for the purposes of climate reconstructions. However, recent dendroecological studies recognize that growth-climate relationships may change due to shifts in climate seasonality, changes in tree size class, tree competition, and possibly due to increases in atmospheric CO2 (Voelker et al. 2006). In theory, higher levels of CO2 in the atmosphere can enhance tree growth by increasing CO2 available for photosynthesis in the leaf, without changing stomatal conductance (gs, the amount of water that moves through the stomata). This results in an increase in plant Water Use Efficiency (WUE), or the amount of carbon taken up per unit of water used, which could help reduce the impacts of drought on trees (McCarroll and Loader 2004). While the effect of atmospheric CO2 on tree growth is still largely debated, past researchers found that Bur Oak (Quercus macrocarpa) trees in Western Minnesota have become less sensitive to drought since the beginning of the 20th century, and mortality due to drought has decreased as well (Wyckoff and Bowers 2009). Additionally, Voelker et al. (2006) found that the positive effects on growth that may result from increased atmospheric CO2 likely decline with tree age. My sampling effort has extended the spatial range of oak sampling in the Midwest, allowing us to test whether a change in the growth–drought relationship over the 20th century is regional and if it has occurred in different oak species and site conditions.
With our data across the Midwest, we find preliminary evidence supporting a change in growth sensitivity to climate. Trees across the Midwest were less sensitive to drought after 1950, and younger trees established under high CO2 were also less sensitive to drought than older trees.
These results are consistent with the previous work in Minnesota (Wyckoff and Bowers 2009), and with a positive enhancement of CO2. In two of the three closed forest sites sampled, we find no difference in the growth-drought sensitivity over time, suggesting that savanna trees, but not forest trees have become less susceptible to drought in the region. While the stand structure (open savanna or closed forest) may help explain where we see shifts in growth-climate sensitivity, species sampled may also play a role, as well as the mean annual precipitation and temperature. To specifically test whether CO2 enhancement is driving the decreased drought sensitivity, I am currently working on a project that tests to see if the composition of carbon isotopes recorded within annual tree rings have changed. The ratio of heavy to light carbon isotopes can be used to quantify plant Water Use Efficiency, which will increase over time if CO2 has a net positive effect on tree growth.
Up Next…
This project is still ongoing and there are several questions that I am still exploring. I am continuing to work on a formal analysis of the tree ring growth data, and look more at species-specific sensitivities to climate, since the preliminary analyses focus on site specific responses.
If growth and sensitivity of growth to climate changes over time, I want to know if it is due to the effects of CO2, or some other factor affecting forest growth. This next year, I will be spending a lot of time in the lab quantifying stable carbon isotopes, to determine if plant WUE increases result in the decrease in drought sensitivity over time.
References:
Charney, N. D., et al. (2016). Observed forest sensitivity to climate implies large changes in 21st century North American forest growth. Ecology Letters, 19(9), 1119–1128. https://doi.org/10.1111/ele.12650
Goring, S. J., et al. (2016). Novel and Lost Forests in the Upper Midwestern United States, from New Estimates of Settlement-Era Composition, Stem Density, and Biomass. PLOS ONE, 11(12), e0151935. https://doi.org/10.1371/journal.pone.0151935
McCarroll, D., & Loader, N. J. (2004). Stable isotopes in tree rings. Quaternary Science Reviews, 23(7–8), 771–801. https://doi.org/10.1016/j.quascirev.2003.06.017
Peterson, D. L. (2014). Climate Change and United Steates Forests. In Climate Change and United States Forests. Springer. Retrieved from http://www.springer.com/us/book/9789400775145
Rhemtulla, J. M., et al. (2007). Regional land-cover conversion in the U.S. upper Midwest: magnitude of change and limited recovery (1850–1935–1993). Landscape Ecology, 22(1), 57–75. https://doi.org/10.1007/s10980-007-9117-3
Voelker, S. L., et al. (2006). Historical CO2 Growth Enhancement Declines with Age in Quercus and Pinus. Ecological Monographs, 76(4), 549–564.
Wyckoff, P. H., & Bowers, R. (2010). Response of the prairie–forest border to climate change: impacts of increasing drought may be mitigated by increasing CO2. Journal of Ecology, 98(1), 197–208. https://doi.org/10.1111/j.1365-2745.2009.01602.x