The Distant Reader can take five different types of input, and this blog posting describes what they are.
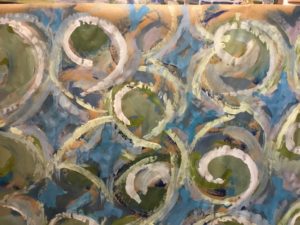
Wall Paper by Eric
The Distant Reader is a tool for reading. It takes an arbitrary amount of unstructured data (text) as input, and it outputs sets of structured data for analysis — reading. Given a corpus of any size, the Distant Reader will analyze the corpus, and it will output a myriad of reports enabling you to use & understand the corpus. The Distant Reader is intended to designed the traditional reading process.
At the present time, the Reader can accept five different types of input, and they include:
- a file
- a URL
- a list of URLs
- a zip file
- a zip file with a companion CSV file
Each of these different types of input are elaborated upon below.
A file
The simplest form of input is a single file from your computer. This can be just about file available to you, but to make sense, the file needs to contain textual data. Thus, the file can be a Word document, a PDF file, an Excel spreadsheet, an HTML file, a plain text file, etc. A file in the form of an image will not work because it contains zero text. Also, not all PDF files are created equal. Some PDF files are only facsimiles of their originals. Such PDF files are merely sets of images concatenated together. In order for PDF files to be used as input, the PDF files need to have been “born digitally” or they need to have had optical character recognition previously applied against them. Most PDF files are born digitally nor do they suffer from being facsimiles.
A good set of use-cases for single file input is the whole of a book, a long report, or maybe a journal article. Submitting a single file to the Distant Reader is quick & easy, but the Reader is designed for analyzing larger rather than small corpora. Thus, supplying a single journal article to the Reader doesn’t make much sense; the use of the traditional reading process probably makes more sense for a single journal article.
A URL
The Distant Reader can take a single URL as input. Given a URL, the Reader will turn into a rudimentary Internet spider and build a corpus. More specifically, given a URL, the Reader will:
- retrieve & cache the content found at the other end of the URL
- extract any URLs it finds in the content
- retrieve & cache the content from these additional URLs
- stop building the corpus but continue with its analysis
In short, given a URL, the Reader will cache the URL’s content, crawl the URL one level deep, cache the result, and stop caching.
Like the single file approach, submitting a URL to the Distant Reader is quick & easy, but there are a number of caveats. First of all, the Reader does not come with very many permissions, and just because you are authorized to read the content at the other end of a URL does not mean the Reader has the same authorization. A lot of content on the Web resides behind paywalls and firewalls. The Reader can only cache 100% freely accessible content.
“Landing pages” and “splash pages” represent additional caveats. Many of the URLs passed around the ‘Net do not point to the content itself, but instead they point to ill-structured pages describing the content — metadata pages. Such pages may include things like authors, titles, and dates, but these things are not presented in a consistent nor computer-readable fashion; they are laid out with aesthetics or graphic design in mind. These pages do contain pointers to the content you want to read, but the content may be two or three more clicks away. Be wary of URLs pointing to landing pages or splash pages.
Another caveat to this approach is the existence of extraneous input due to navigation. Many Web pages include links for navigating around the site. They also include links to things like “contact us” and “about this site”. Again, the Reader is sort of stupid. If found, the Reader will crawl such links and include their content in the resulting corpus.
Despite these drawbacks there are number of excellent use-cases for single URL input. One of the best is Wikipedia articles. Feed the Reader a URL pointing to a Wikipedia article. The Reader will cache the article itself, and then extract all the URLs the article uses as citations. The Reader will then cache the content of the citations, and then stop caching.
Similarly, a URL pointing to an open access journal article will function just like the Wikipedia article, and this will be even more fruitful if the citations are in the form of freely accessible URLs. Better yet, consider pointing the Reader to the root of an open access journal issue. If the site is not overly full of navigation links, and if the URLs to the content itself are not buried, then the whole of the issue will be harvested and analyzed.
Another good use-case is the home page of some sort of institution or organization. Want to know about Apple Computer, the White House, a conference, or a particular department of a university? Feed the root URL of any of these things to the Reader, and you will learn something. At the very least, you will learn how the organization prioritizes its public face. If things are more transparent than not, then you might be able to glean the names and addresses of the people in the organization, the public policies of the organization, or the breadth & depth of the organization.
Yet another excellent use-case includes blogs. Blogs often contain content at their root. Navigations links abound, but more often than not the navigation links point to more content. If the blog is well-designed, then the Reader may be able to create a corpus from the whole thing, and you can “read” it in one go.
A list of URLs
The third type of input is a list of URLs. The list is expected to be manifested as a plain text file, and each line in the file is a URL. Use whatever application you desire to build the list, but save the result as a .txt file, and you will probably have a plain text file.‡
Caveats? Like the single URL approach, the list of URLs must point to freely available content, and pointing to landing pages or splash pages is probably to be avoided. Unlike the single URL approach, the URLs in the list will not be used as starting points for Web crawling. Thus, if the list contains ten items, then ten items will be cached for analysis.
Another caveat is the actual process of creating the list; I have learned that is actually quite difficult to create lists of URLs. Copying & pasting gets old quickly. Navigating a site and right-clicking on URLs is tedious. While search engines & indexes often provide some sort of output in list format, the lists are poorly structured and not readily amenable to URL extraction. On the other hand, there are more than a few URL extraction tools. I use a Google Chrome extension called Link Grabber. [1] Install Link Grabber. Use Chrome to visit a site. Click the Link Grabber button, and all the links in the document will be revealed. Copy the links and paste them into a document. Repeat until you get tired. Sort and peruse the list of links. Remove the ones you don’t want. Save the result as a plain text file.‡ Feed the result to the Reader.
Despite these caveats, the list of URLs approach is enormously scalable; the list of URLs approach is the most scalable input option. Given a list of five or six items, the Reader will do quite well, but the Reader will operate just as well if the list contains dozens, hundreds, or even thousands of URLs. Imagine reading the complete works of your favorite author or the complete run of an electronic journal. Such is more than possible with the Distant Reader.‡
A zip file
The Distant Reader can take a zip file as input. Create a folder/directory on your computer. Copy just about any file into the folder/directory. Compress the file into a .zip file. Submit the result to the Reader.
Like the other approaches, there are a few caveats. First of all, the Reader is not able to accept .zip files whose size is greater than 64 megabytes. While we do it all the time, the World Wide Web was not really designed to push around files of any great size, and 64 megabytes is/was considered plenty. Besides, you will be surprised how many files can fit in a 64 megabyte file.
Second, the computer gods never intended file names to contain things other than simple Romanesque letters and a few rudimentary characters. Now-a-days our file names contain spaces, quote marks, apostrophes, question marks, back slashes, forward slashes, colons, commas, etc. Moreover, file names might be 64 characters long or longer! While every effort as been made to accomodate file names with such characters, your milage may vary. Instead, consider using file names which are shorter, simpler, and have some sort of structure. An example might be first word of author’s last name, first meaningful word of title, year (optional), and extension. Herman Melville’s Moby Dick might thus be named melville-moby.txt. In the end the Reader will be less confused, and you will be more able to find things on your computer.
There are a few advantages to the zip file approach. First, you can circumvent authorization restrictions; you can put licensed content into your zip files and it will be analyzed just like any other content. Second, the zip file approach affords you the opportunity to pre-process your data. For example, suppose you have downloaded a set of PDF files, and each page includes some sort of header or footer. You could transform each of these PDF files into plain text, use some sort of find/replace function to remove the headers & footers. Save the result, zip it up, and submit it to the Reader. The resulting analysis will be more accurate.
There are many use-cases for the zip file approach. Masters and Ph.D students are expected to read large amounts of material. Save all those things into a folder, zip them up, and feed them to the Reader. You have been given a set of slide decks from a conference. Zip them up and feed them to the Reader. A student is expected to read many different things for History 101. Download them all, put them in a folder, zip them up, and submit them to the Distant Reader. You have written many things but they are not on the Web. Copy them to a folder, zip them up, and “read” them with the… Reader.
A zip file with a companion CSV file
The final form of input is a zip file with a companion comma-separated value (CSV) file — a metadata file.
As the size of your corpus increases, so does the need for context. This context can often be manifested as metadata (authors, titles, dates, subject, genre, formats, etc.). For example, you might want to compare & contrast who wrote what. You will probably want to observe themes over space & time. You might want to see how things differ between different types of documents. To do this sort of analysis you will need to know metadata regarding your corpus.
As outlined above, the Distant Reader first creates a cache of content — a corpus. This is the raw data. In order to do any analysis against the corpus, the corpus must be transformed into plain text. A program called Tika is used to do this work. [2] Not only does Tika transform just about any file into plain text, but it also does its best to extract metadata. Depending on many factors, this metadata may include names of authors, titles of documents, dates of creation, number of pages, MIME-type, language, etc. Unfortunately, more often than not, this metadata extraction process fails and the metadata is inaccurate, incomplete, or simply non-existent.
This is where the CSV file comes in; by including a CSV file named “metadata.csv” in the .zip file, the Distant Reader will be able to provide meaningful context. In turn, you will be able to make more informed observations, and thus your analysis will be more thorough. Here’s how:
- assemble a set of files for analysis
- use your favorite spreadsheet or database application to create a list of the file names
- assign a header to the list (column) and call it “file”
- create one or more columns whose headers are “author” and/or “title” and/or “date”
- to the best of your ability, update the list with author, title, or date values for each file
- save the result as a CSV file named “metadata.csv” and put it in the folder/directory to be zipped
- compress the folder/directory to create the zip file
- submit the result to the Distant Reader for analysis
The zip file with a companion CSV file has all the strengths & weakness of the plain o’ zip file, but it adds some more. On the weakness side, creating a CSV file can be both tedious and daunting. On the other hand, many search engines & index export lists with author, title, and data metadata. One can use these lists as the starting point for the CSV file.♱ On the strength side, the addition of the CSV metadata file makes the Distant Reader’s output immeasurably more useful, and it leads the way to additional compare & contrast opportunities.
Summary
To date, the Distant Reader takes five different types of input. Each type has its own set of strengths & weaknesses:
- a file – good for a single large file; quick & easy; not scalable
- a URL – good for getting an overview of a single Web page and its immediate children; can include a lot of noise; has authorization limitations
- a list of URLs – can accomodate thousands of items; has authorization limitations; somewhat difficult to create list
- a zip file – easy to create; file names may get in the way; no authorization necessary; limited to 64 megabytes in size
- a zip file with CSV file – same as above; difficult to create metadata; results in much more meaningful reports & opportunities
Happy reading!
Notes & links
‡ Distant Reader Bounty #1: To date, I have only tested plain text files using line-feed characters as delimiters, such are the format of plain text files in the Linux and Macintosh worlds. I will pay $10 to the first person who creates a plain text file of URLs delimited by carriage-return/line-feed characters (the format of Windows-based text files) and who demonstrates that such files break the Reader. “On you mark. Get set. Go!”
‡ Distant Reader Bounty #2: I will pay $20 to the first person who creates a list of 2,000 URLs and feeds it to the Reader.
♱ Distant Reader Bounty #3: I will pay $30 to the first person who writes a cross-platform application/script which successfully transforms a Zotero bibliography into a Distant Reader CSV metadata file.
[1] Link Grabber – http://bit.ly/2mgTKsp
[2] Tika – http://tika.apache.org