The majority of people know what a fall is and, in fact, many people have unfortunately experienced one or a few. But what would be a good definition for what a fall is? Simply put, a fall is something that happens when you lose your balance and cannot recover. Falls have the potential to ruin anyone’s day. For some, however, the risk is far more severe than that as falls are one of the leading factors in injury and death among the elderly population. This will continue to be a problem as the number of elderly people in the United States is expected to increase dramatically over the next fifty years.

There are a few reasons that make the elderly a more at-risk population. A major reason is the reduced reaction time that occurs as people age. Another reason is due to the reduction in bone density as people age. Additionally, some elderly people may suffer from disorders such as Parkinson’s Disease or other neurocognitive issues that cause diminished motor control, leading to more instability and a higher chance of falling. Parkinson’s Disease or (PD) is a nervous system disorder that affects both cognitive and motor functions. In PD patients, increased falling is often linked with cognitive decline according to this paper by Fasano, et al. While there is not much research on PD and falls, one benefit is that fall rate could be used as an early predictor of PD in individuals as injuries stemming from falls have the potential to be higher than normal for people even ten years before the onset of Parkinson’s Disease.

The best way to reduce fall risks is to prevent falls. A study by Hahn and Chou attempted to predict fall risk in individuals using a system similar to machine learning called a neural network to evaluate a group of subjects and place them into different groups based on level of fall risk. By categorizing the fall risk of individuals, one can know if they are likely to suffer from falls and can take appropriate measures to prevent them. The subjects of this study were split into two groups classified as healthy, with no preexisting conditions, and fallers, with self-reported issues. Each subject participated in three walking trials and different measurements were recorded including gait measurements, center of mass movement, and muscle response. The first distinction made was to classify each participant as either healthy or as a faller. After finding a baseline for healthy subjects, the participants were further classified by level of fall risk from very low risk to very high risk. This neural network had about ninety percent accuracy in separating healthy patients from ‘fallers’ and was successful in sorting by risk level.
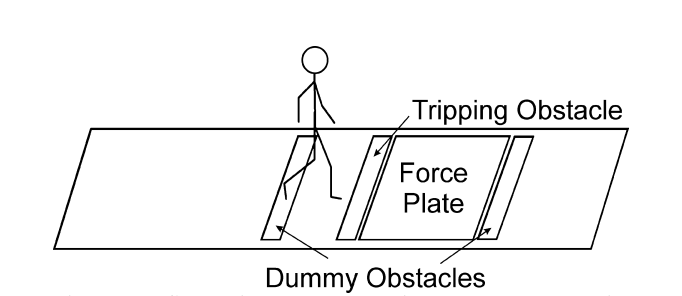
Another way to reduce the risk of falls is to reduce the severity of them. This study by Bieryla, et al. contained twelve healthy subjects and was designed to test the application of motor learning to reduce fall risk. It was conducted as a pretest/posttest study where the participants were divided into two groups: a control group and an experimental group. A first trial was performed where a trip was simulated using obstacles placed on a treadmill as shown in the figure. The participants were in a harness and were told to attempt to recover from the trip incident. The dummy obstacles were images that appeared to be obstacles and were used to ensure the validity of the results. After this first trial, the control group spent time walking on the treadmill while the experimental group underwent trip recovery training. The recovery training consisted of practicing stepping over an obstacle that resembled the motion of regaining balance after tripping. Then, a second trial was performed to see the effect of the recovery training. Overall, the results were positive and they showed beneficial effects of the trip recovery training.
Featured image from Vaartkapoen by Tom Frantzen imaged by Trougnouf licensed under CC BY 4.0.